How companies can get started with AI The program for our Artificial Intelligence Conference in London is structured to help companies that are still very much in the early stages of AI adoption. Judging by the list of countries putting out policy papers on AI and automation technologies there is very strong interest in AI across the globe. In order to asses the current state of readiness across regions we recently conducted a survey (full report forthcoming) of the state of adoption of machine learning tools and technologies (a lot of what is being currently described as AI is really ML). The survey yielded 11400 respondents including 2000 respondents from Europe: As we assembled the program for our inaugural Artificial Intelligence Conference in London this October we recognized that many companies and organizations around the world are still very much in the early stages of adoption. Anyone wanting to get started on AI technologies will have to wade through an array of methods and technologies many of which are still very much on the leading edge. The good news is that some companies at the forefront are beginning to share best practices tools and lessons learned as they deploy AI technologies. In this short post Ill use key portions of our AI conference in London to describe how companies may want to get started in AI. AI in the Enterprise: Best practices Has machine learning impacted how companies organize and build teams? We found that as companies gain more experience with machine learning they are more likely to start hiring and attracting specialists including data scientists research scientists and machine learning engineers. But there are many more decisions companies need to make on the path to embracing AI. Organizations must work through a series of assessments in order to successfully deploy AI and automation technologies: What are the key technologies (hardware and software) and what are their limitations? How much data does one need to use these technologies? Where can I get data to augment my existing data sets? How does one organize and hire for AI? What types of problems or tasks lend themselves to automation? What are some initial use cases one can consider? How do you maintain momentum and build upon the lessons learned from previous projects? We put together training programs tutorials and sessions designed to help attendees understand how to move forward with best practices tools and technologies used at many leading companies. “AI in the Enterprise” AI for managers (2-day training) Bringing AI into the enterprise (1-day tutorial) Executive Briefing: Moving AI Off Your Product Roadmap and Into Your Products Executive Briefing: Organizational Design For Effective AI Early applications of AI technologies As I noted earlier much of the current excitement around AI pertains to recent progress in machine learningspecifically deep learning and reinforcement learning. Both of these class of methods impact existing products and data types (text temporal and structured data) and also enable companies to unlock new data sources (including audio video and images). Progress in automating enterprise workflows will depend on foundational technologies (hardware and software) and breakthroughs in areas like computer vision natural language understanding and generation and speech technologies. We are beginning to see many interesting enterprise applications of both deep learning and reinforcement learning particularly in computer vision and text but also in areas where many enterprises already had analytic solutions in place (recommenders and forecasting tools). “Reinforcement Learning” Building reinforcement learning applications with Ray (tutorial) “Computer Vision” “Temporal data and time-series” “Text Language and Speech” “Deep Learning models” Implementing AI AI applications rely on machine learning models as well as hardware and software infrastructure for model training deployment and management. Machine learning itself requires robust end-to-end data pipelines spanning data ingestion data preparation and data management. Depending on the nature of the application a knowledge base or graph components for reasoning and planning simulation platforms and user interfaces might also come into play. For our upcoming AI conference in London we assembled sessions on many of core components in an AI technology stack. We have content ranging from tutorials on how to use specific libraries and technologies to how to launch data markets and networks to sessions on best practices for building and architecting AI applications. “Edge computing and Hardware” “Platforms and infrastructure” “Deep Learning Tools” and “Reinforcement Learning Tools” “Interfaces and UX” “Data Networks and Data Markets” Case studies Among the many the challenges faced by organizations is identifying good use cases for AI and automation technologies. One of our main goals for this conference series is to foster a community of professionals interested in building and using AI applications. To that end we put together a series of sessions where companies describe how they put AI technologies to work within their organizations. We are also planning a series of attendee networking events at the conference. Heres a sampling of sessions from a few domains: “Financial Services” “Media Marketing Advertising” “Retail and e-commerce” Ethics privacy security As I noted in a recent post there is growing awareness among major stakeholders about the importance of data privacy ethics and security. In our recent ML adoption survey we found that respondents are starting to engage and they are beginning to take into account factors such as bias transparency and privacy in their machine learning systems. Fairness and transparency and privacy-preserving analytics have become areas of active academic research but its important to emphasize that tools and best practices are just beginning to emerge. These concerns cut across geographic regions and industries but with the launch of GDPR Europe stands out for taking a leadership position in data privacy. AI policy papers from several European countries also emphasize the need for fairness and transparency in machine learning and AI. With all these considerations in mind for our inaugural Artificial Intelligence Conference in London we have assembled an outstanding series of presentations on the practical aspects of incorporating ethics privacy and security into AI systems. “Ethics Privacy and Security” Executive Briefing: Ethical AI – How To Build Products that Customers Will Love and Trust Data Privacy and its implications for Deep Learning How CLEVER is your neural network? Robustness evaluation against adversarial examples Continue reading How companies can get started with AI. ift.tt/2LXzpAq
- /
- /Akanksha garg
- /No Comment
- /2455 views
- /artificial intelligence (ai)artificial intelligence (ai) and machine learning companiesartificial intelligence (ai) and machine learning courseartificial intelligence (ai) applications for covid-19 pandemicartificial intelligence (ai) engineerartificial intelligence (ai) in software testingartificial intelligence (ai) solution in azureartificial intelligence (ai) than conventional programmingcompaniesstarted
How companies can get started with AI
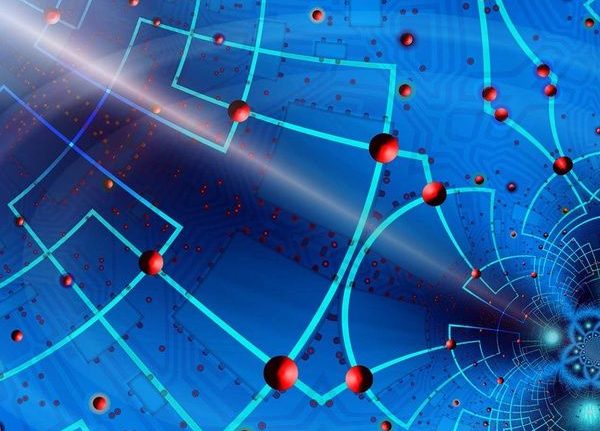
Tags:artificial intelligence (ai)artificial intelligence (ai) and machine learning companiesartificial intelligence (ai) and machine learning courseartificial intelligence (ai) applications for covid-19 pandemicartificial intelligence (ai) engineerartificial intelligence (ai) in software testingartificial intelligence (ai) solution in azureartificial intelligence (ai) than conventional programmingcompaniesstarted
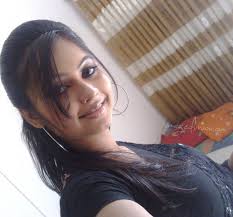
Akanksha garg
she is Experienced Writer/Editor/Logo Designer.For over 6 years I have worked as a writer and editor for magazines, books, and online forums such as websites, blogs, and e-books. Whether writing my own thoughts or perfecting those written by others, I communicate passionately and work diligently.